Shen Yan
shenyan at google dot com
I am a Research Scientist at Google DeepMind, where I work on video-text modeling and its applications.
I did my PhD at the Computer Science Department at Michigan State University. During my PhD, I was fortunate to work with people at Bosch Research, Bytedance AML, Abacus.AI, Argo AI, and Google Research (Perception and Brain team).
Google Scholar /
LinkedIn /
Twitter
|
|
|
VideoPrism: A Foundational Visual Encoder for Video Understanding
Google Research
ICML, 2024
blog /
arXiv /
bibtex
A general-purpose video encoder that tackles diverse video understanding tasks with a single frozen model.
|
|
Pixel Aligned Language Models
Jiarui Xu,
Xingyi Zhou,
Shen Yan,
Xiuye Gu,
Anurag Arnab,
Chen Sun,
Xiaolong Wang,
Cordelia Schmid
CVPR, 2024
arXiv /
code /
bibtex
We propose PixelLLM to equip LLMs with pixel-aligned localization capability.
|
|
Streaming Dense Video Captioning
Xingyi Zhou,
Anurag Arnab,
Shyamal Buch,
Shen Yan,
Austin Myers,
Xuehan Xiong,
Arsha Nagrani,
Cordelia Schmid
CVPR, 2024
arXiv /
code /
bibtex
An online video captioner based on token clustering and streaming decoding.
|
|
UnLoc: A Unified Framework for Video Localization Tasks
Shen Yan*,
Xuehan Xiong*,
Arsha Nagrani,
Anurag Arnab,
Zhonghao Wang,
Weina Ge,
David Ross,
Cordelia Schmid
ICCV, 2023
arXiv /
code /
bibtex
UnLoc unifies moment retrieval, temporal localization and action segmentation with a single stage model.
|
|
VideoCoCa: Video-Text Modeling with Zero-Shot Transfer from Contrastive Captioners
Shen Yan*,
Tao Zhu*,
Zirui Wang,
Yuan Cao,
Mi Zhang,
Soham Ghosh,
Yonghui Wu,
Jiahui Yu
arXiv, 2023
arXiv /
bibtex
VideoCoCa maximally reuses pretrained CoCa and minimizes additional training cost.
|
|
Soft Augmentation for Image Classification
Yang Liu,
Shen Yan,
Laura Leal-Taixé,
James Hays,
Deva Ramanan
CVPR, 2023  
arXiv /
code /
bibtex
Soft augmentations produce better calibrated models on occluded examples.
|
|
Multiview Transformers for Video Recognition
Shen Yan,
Xuehan Xiong,
Anurag Arnab,
Zhichao Lu,
Mi Zhang,
Chen Sun,
Cordelia Schmid
CVPR, 2022  
arXiv /
code /
bibtex
A simple method for capturing multiresolution temporal context in transformers.
|
|
Deep AutoAugment
Yu Zheng,
Zhi Zhang,
Shen Yan,
Mi Zhang
ICLR, 2022  
arXiv /
code /
bibtex /
slides
Build a data augmentation policy progressively based on regularized gradient matching.
|
|
NAS-Bench-x11 and the Power of Learning Curves
Shen Yan*,
Colin White*,
Yash Savani,
Frank Hutter
NeurIPS, 2021  
arXiv /
code /
bibtex /
slides
A surrogate method to create multi-fidelity NAS benchmarks.
|
|
CATE: Computation-aware Neural Architecture Encoding with Transformers
Shen Yan,
Kaiqiang Song,
Fei Liu,
Mi Zhang
ICML, 2021   (Long Presentation)
video: 17 min/
arXiv /
code /
bibtex
Pre-training computation-aware architecture embeddings can also help with architecture search.
|
|
Does Unsupervised Architecture Representation Learning Help Neural Architecture Search?
Shen Yan,
Yu Zheng,
Wei Ao,
Xiao Zeng,
Mi Zhang
NeurIPS, 2020  
video: 3 min/
arXiv /
code /
bibtex
Pre-training structure-aware architecture embeddings help architecture search.
|
|
MutualNet: Adaptive ConvNet via Mutual Learning from Network Width and Resolution
Taojiannan Yang,
Sijie Zhu,
Chen Chen,
Shen Yan,
Mi Zhang,
Andrew Wills
ECCV, 2020   (Oral)
video: 10 min/
arXiv /
code /
bibtex
Mutual learning with input resolution and network width improves accuracy-efficiency tradeoffs.
|
|
Improve Unsupervised Domain Adaptation with Mixup Training
Shen Yan,
Huan Song,
Nanxiang Li,
Lincan Zou,
Liu Ren
arXiv, 2020
arXiv /
code /
bibtex
Mixup can help with unsupervised domain adaptation.
|
|
Deep Fisher Faces
Harald Hanselmann,
Shen Yan,
Hermann Ney
BMVC, 2017
bibtex
We extend the center loss with an inter-class loss reminiscent of the popular early face recognition approach Fisherfaces.
|
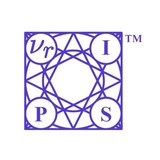 |
PC member, AutoML Workshop, ICML 2021
PC member, NAS Workshop, ICLR 2021
Reviewer, ICML 2020, 2021, 2022, 2023
Reviewer, ICLR 2021, 2022, 2023
Reviewer, NeurIPS 2020, 2021, 2022, 2023
Reviewer, CVPR 2021, 2022, 2023
Reviewer, ICCV 2021, 2023
Reviewer, ECCV 2022
Reviewer, TMLR 2022, 2023
Reviewer, PAMI 2022, 2023
|
|
TA for Bachelor, Kinect Programming, Fall 2015
|
|